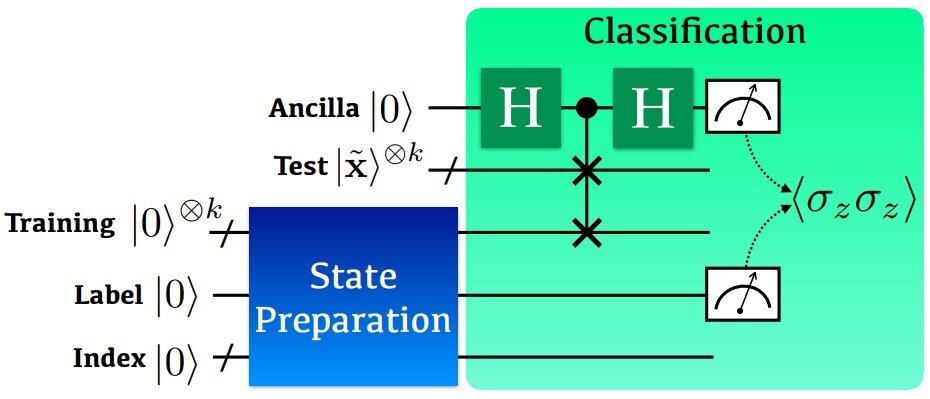
The research team from the Korea Advanced Institute of Science and Technology (KAIST) have introduced a new method for machine-learning classifications in quantum computing. They proposed a quantum classifier based on quantum state fidelity by using a different initial state and replacing the Hadamard classification with a swap test.
Unlike the conventional approach, this method is expected to significantly enhance the classification tasks when the training dataset is small, by exploiting the quantum advantage in finding non-linear features in a large feature space.
The idea of the classification algorithm with a nonlinear kernel is that given a quantum test state, the protocol calculates the weighted power sum of the fidelities of quantum data in quantum parallel via a swap-test circuit followed by two single-qubit measurements. This requires only a small number of quantum data operations regardless of the size of data. The novelty of this approach lies in the fact that labeled training data can be densely packed into a quantum state and then compared to the test data.
The team implemented the classifier and compared classical simulations using the five-qubit IBM quantum computer that is freely available to public users via cloud service. (Phys.org)
The paper has been published in npj Quantum Information.