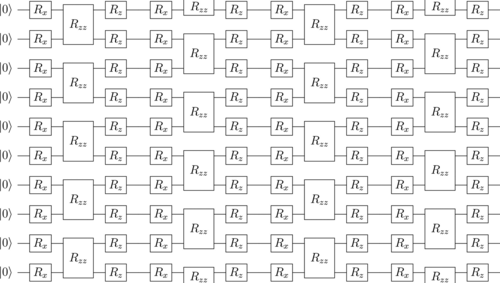
Skoltech scientists have shown that quantum enhanced machine learning can be used on quantum (as opposed to classical) data, overcoming a significant slowdown common to these applications and opening a “fertile ground to develop computational insights into quantum systems.”
The standard approach to quantum enhanced machine learning has been to apply quantum algorithms to classical data. In other words, classical data (represented by bit strings of 1’s and 0’s) must be stored or otherwise represented by a quantum processor before quantum effects can be utilized. This is called the data-readin problem. Data-readin serves to limit the speedup that is possible using quantum enhanced machine learning algorithms.
The team has merged quantum enhanced machine learning with quantum enhanced simulation, applying their approach to study phase transitions in many-body quantum magnetic problems. In doing so, they train quantum neural networks using only quantum states as data. In other words, the authors circumvent the data-readin problem by feeding in quantum mechanical states of matter. Such states appear to generally require an impossible amount of memory to represent using standard (non-quantum) approaches.
The work uses a subroutine known as the Variational Quantum Eigensolver (VQE) which is an algorithm that iteratively finds an approximation to the ground state of a given quantum Hamiltonian. The output of this subroutine is a set of instructions to prepare a quantum state on a quantum computer. (Phys.org)
The paper was published in the journal Physical Review A.