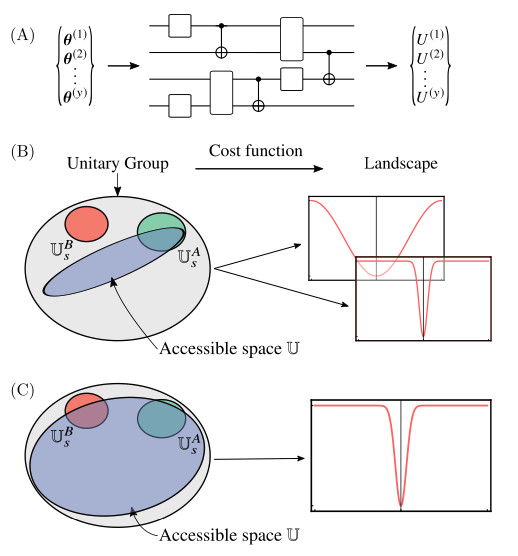
Researchers at Los Alamos National Laboratory proved a fundamental theorem for Quantum Neural Networks (QNNs), or more generally, for any parameterized quantum circuit (often called an “ansatz”).
Parameterized quantum circuits serve as ansätze for solving variational problems and provide a flexible paradigm for programming NISQ computers. In Variational Quantum Algorithms (VQAs), a problem-specific cost function is efficiently evaluated on a quantum computer, while a classical optimizer trains a parameterized quantum circuit to minimize this cost.
Ideally, such ansätze should be highly expressive so that a close approximation of the desired solution can be accessed. On the other hand, the ansatz must also have sufficiently large gradients to allow for training.
The team has derived a fundamental relationship between these two essential properties: expressibility and trainability. This is done by extending the well established barren plateau phenomenon, which holds for ansätze that form exact 2-designs, to arbitrary ansätze.
Specifically, they calculated the variance in the cost gradient in terms of the expressibility of the ansatz, as measured by its distance from being a 2- design. Their resulting bounds indicate that highly expressive ansätze exhibit flatter cost landscapes and therefore will be harder to train.