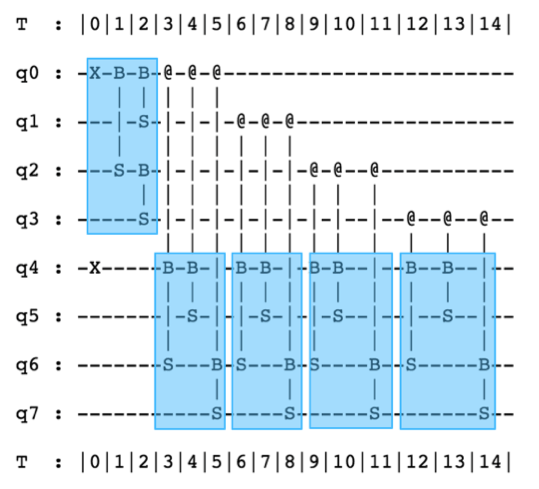
QC Ware and IonQ have collaborated and run experiment that demonstrated Machine Learning on near-term quantum computers can achieve the same or better level of accuracy and can aspire to be faster than on classical computers.
QC Ware is known to be involved in Quantum Machine Learning.
QC Ware and IonQ teams ran QC Ware’s machine learning algorithm for classification on IonQ’s 11 qubit system. They overcame what was an insurmountable hurdle in QML — loading classical data onto quantum states in a noise-resilient way to allow efficient and robust QML applications. Central to the success of their experiment are QC Ware’s own Forge Data Loader™ technology, which optimally transforms classical data onto quantum states, and the high-quality, fully connected qubits of IonQ’s 11 qubit trapped-ion quantum machine.
The team designed a quantum Nearest Centroid classifier, using techniques for efficiently loading classical data into quantum states and performing distance estimations, and experimentally demonstrate it, matching the accuracy of classical nearest centroid classifiers for the MNIST handwritten digits dataset and achieving up to 100% accuracy for 8-dimensional synthetic data.
The paper can be read there.